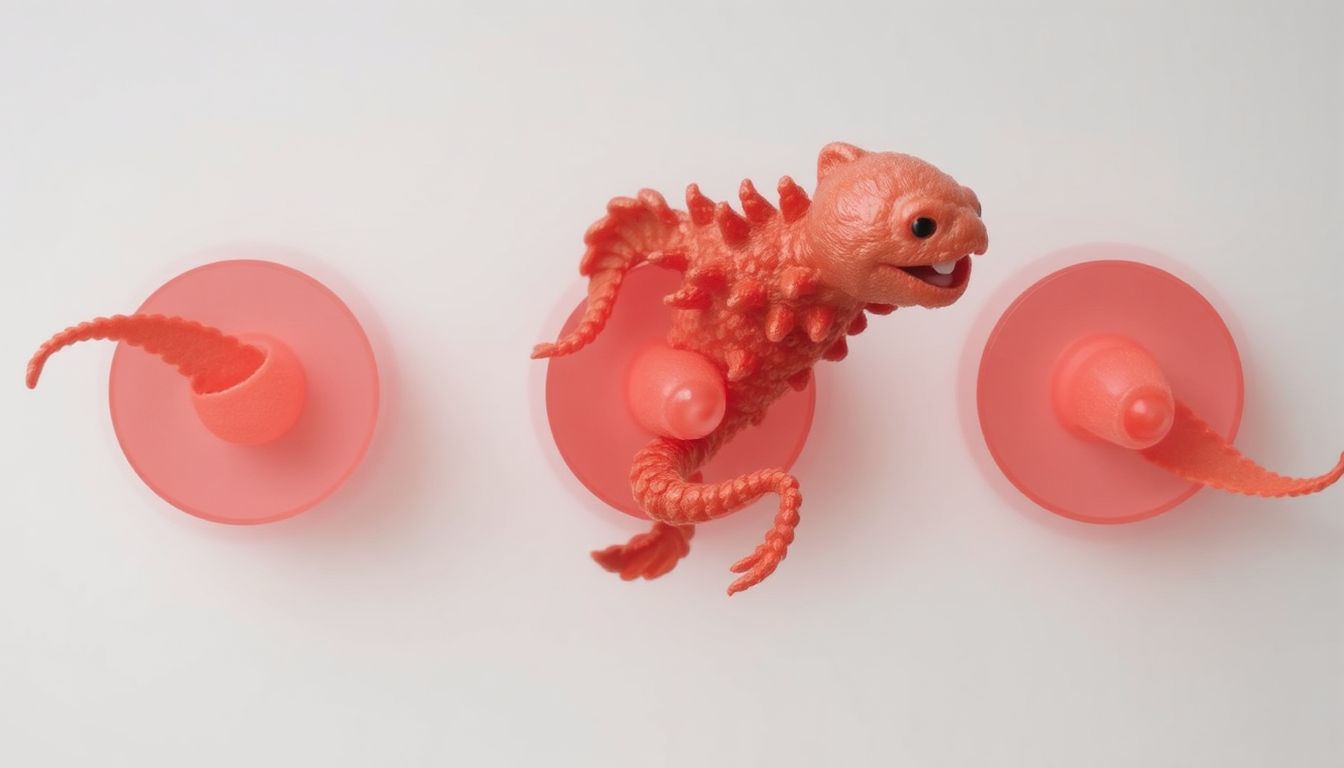
Introduction to Alibaba Qwen QwQ-32B
Artificial intelligence continues to evolve at a rapid pace, and Alibaba's Qwen team has once again pushed the boundaries with their latest innovation: the QwQ-32B. This 32 billion parameter AI model is a testament to the power of scaled reinforcement learning (RL), demonstrating performance that rivals even larger models. In this blog post, we’ll dive deep into what makes QwQ-32B a game-changer, how it leverages RL, and why it’s a significant step forward in AI development.
What is Scaled Reinforcement Learning?
Reinforcement learning (RL) is a type of machine learning where an AI agent learns to make decisions by performing actions and receiving feedback from its environment. Traditionally, RL has been used in areas like robotics, gaming, and recommendation systems. However, scaling RL to larger models like QwQ-32B opens up new possibilities for enhancing reasoning, problem-solving, and adaptability in AI systems.
Scaling RL involves training models on vast amounts of data and computational resources, enabling them to generalize better and perform complex tasks. The Qwen team has successfully integrated RL into QwQ-32B, allowing it to think critically, use tools, and adapt its reasoning based on environmental feedback. This approach has proven to be a game-changer, as it bridges the gap between model size and performance.
QwQ-32B: A Closer Look
QwQ-32B is a 32 billion parameter AI model that has been pretrained on extensive world knowledge. What sets it apart is its ability to achieve performance comparable to models with significantly more parameters, such as DeepSeek-R1, which boasts 671 billion parameters. This is a remarkable achievement and highlights the effectiveness of RL when applied to robust foundation models.
The model has been evaluated across a range of benchmarks, including AIME24, LiveCodeBench, LiveBench, IFEval, and BFCL. These benchmarks assess its mathematical reasoning, coding proficiency, and general problem-solving capabilities. Let’s take a closer look at how QwQ-32B performed in these tests.
Benchmark Results
Here’s a breakdown of QwQ-32B’s performance across key benchmarks:
- AIME24: QwQ-32B achieved a score of 79.5, slightly behind DeepSeek-R1’s 79.8 but significantly ahead of other models like OpenAl-o1-mini, which scored 63.6.
- LiveCodeBench: QwQ-32B scored 63.4, closely matched by DeepSeek-R1’s 65.9, and outperforming other distilled models and OpenAl-o1-mini’s 53.8.
- LiveBench: QwQ-32B achieved 73.1, with DeepSeek-R1 scoring 71.6, and outperforming other models like OpenAl-o1-mini, which scored 57.5.
- IFEval: QwQ-32B scored 83.9, very close to DeepSeek-R1’s 83.3, and leading other models like OpenAl-o1-mini, which scored 59.1.
- BFCL: QwQ-32B achieved 66.4, with DeepSeek-R1 scoring 62.8, demonstrating a clear lead over other models.
These results highlight QwQ-32B’s exceptional performance across a variety of tasks, showcasing its versatility and adaptability.
The Role of Reinforcement Learning in QwQ-32B
The Qwen team’s approach to scaling RL involved a multi-stage process. The initial stage focused on math and coding tasks, utilizing accuracy verifiers and code execution servers. The second stage expanded to general capabilities, incorporating rewards from general reward models and rule-based verifiers.
This multi-stage RL process allowed QwQ-32B to enhance its reasoning capabilities without compromising performance in other areas. As the team explained, “We find that this stage of RL training with a small amount of steps can increase the performance of other general capabilities, such as instruction following, alignment with human preference, and agent performance, without significant performance drop in math and coding.”
Cold-Start Checkpoint and Outcome-Based Rewards
One of the key innovations in QwQ-32B’s development was the use of a cold-start checkpoint and outcome-based rewards. The cold-start checkpoint allowed the model to begin training from a robust foundation, while outcome-based rewards ensured that the model received feedback based on the quality of its actions.
This approach not only improved the model’s performance but also made the training process more efficient. By focusing on specific tasks and gradually expanding to general capabilities, the Qwen team was able to achieve remarkable results with QwQ-32B.
Open-Weight Model and Accessibility
One of the most exciting aspects of QwQ-32B is its accessibility. The model is open-weight and available on platforms like Hugging Face and ModelScope under the Apache 2.0 license. This means that developers and researchers can freely access and experiment with the model, further advancing the field of AI.
Additionally, QwQ-32B is accessible via Qwen Chat, making it easier for users to interact with the model and explore its capabilities. The Qwen team views this as an initial step in scaling RL to enhance reasoning capabilities and aims to further explore the integration of agents with RL for long-horizon reasoning.
The Future of AI with QwQ-32B
The development of QwQ-32B represents a significant milestone in the journey toward Artificial General Intelligence (AGI). By combining robust foundation models with scaled reinforcement learning, the Qwen team has demonstrated the potential to enhance reasoning, problem-solving, and adaptability in AI systems.
As the team stated, “As we work towards developing the next generation of Qwen, we are confident that combining stronger foundation models with RL powered by scaled computational resources will propel us closer to achieving AGI.”
Conclusion
Alibaba’s Qwen QwQ-32B is a groundbreaking AI model that showcases the power of scaled reinforcement learning. With its impressive performance across a range of benchmarks and its accessibility to developers and researchers, QwQ-32B is poised to make a significant impact on the field of AI. As we continue to explore the potential of RL and other advanced techniques, models like QwQ-32B will play a crucial role in shaping the future of artificial intelligence.