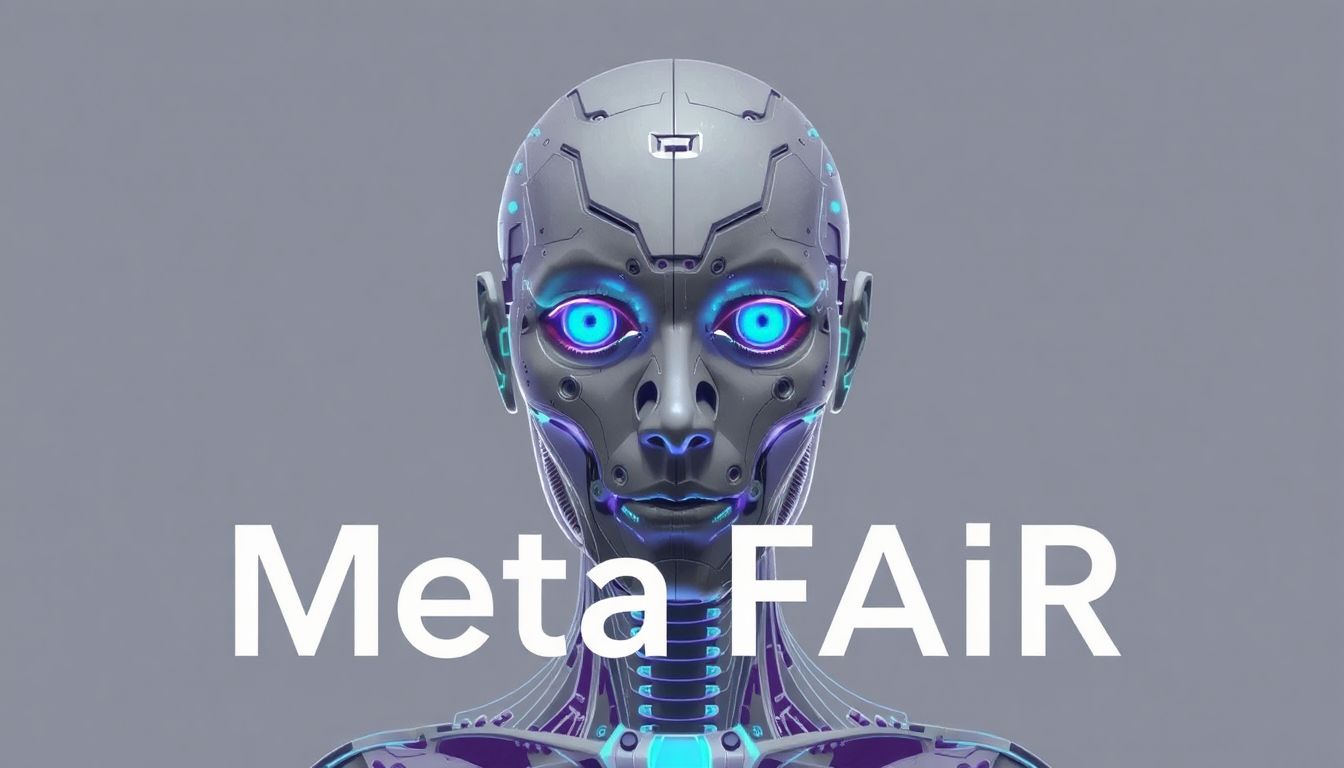
Meta FAIR Advances Human-Like AI with Five Major Releases
Artificial intelligence is evolving at a breakneck pace, and Meta's Fundamental AI Research (FAIR) team is at the forefront of this revolution. The team recently unveiled five groundbreaking projects that bring us closer to creating AI systems with human-like intelligence. These releases span vision, language, robotics, and collaborative reasoning—each pushing the boundaries of what machines can perceive, understand, and accomplish.
Why These Releases Matter
Meta's goal isn't just about making AI smarter—it's about making it more intuitive, adaptable, and capable of interacting with the world the way humans do. Whether it's recognizing subtle details in images, understanding complex language queries, or collaborating seamlessly with people, these advancements represent significant strides toward truly intelligent machines.
1. The Perception Encoder: AI That Sees Like a Human
Imagine an AI that doesn't just "see" an image but understands it with the nuance of a human observer. That's the promise of Meta's Perception Encoder, a state-of-the-art vision model designed to interpret visual data with unprecedented accuracy.
What Makes It Special?
Traditional vision models struggle with fine details or complex scenes, but the Perception Encoder excels in zero-shot classification (identifying objects it wasn't explicitly trained on) and retrieval tasks. Whether it's spotting a camouflaged stingray in the ocean or identifying a rare bird in a cluttered background, this model outperforms existing open-source and proprietary systems.
But it doesn't stop at images—it extends its prowess to videos and even enhances language models when combined with them. In tests, it improved performance on visual question answering, spatial reasoning, and even understanding camera movements relative to objects.
2. Perception Language Model (PLM): Bridging Vision and Language
If the Perception Encoder is the eyes, the Perception Language Model (PLM) is the brain that connects what's seen with what's understood. This open-source model is designed to tackle complex vision-language tasks without relying on proprietary data, making it a valuable resource for researchers.
Key Features of PLM
Meta trained PLM using a mix of synthetic data and open datasets, ensuring transparency. To address gaps in video understanding, they also introduced a massive new dataset—2.5 million human-labeled samples focused on fine-grained video question answering and spatio-temporal captioning.
Available in 1B, 3B, and 8B parameter versions, PLM caters to different research needs. Alongside the model, Meta released PLM-VideoBench, a benchmark designed to test AI on fine-grained activity understanding—something many existing benchmarks miss.
3. Meta Locate 3D: Giving Robots Spatial Awareness
Robots navigating real-world environments need more than just sensors—they need to understand spatial relationships based on natural language. That's where Meta Locate 3D comes in.
How It Works
This model processes 3D point clouds from depth-sensing cameras and interprets open-vocabulary commands like "flower vase near TV console." Unlike simpler systems, it considers context and relationships between objects to pinpoint the correct item.
The model consists of three parts: preprocessing 2D features into 3D point clouds, encoding them with a pretrained 3D-JEPA model, and decoding the query to produce precise bounding boxes and masks.
To support development, Meta also released a new dataset with 130,000 language annotations across 1,346 scenes, doubling existing resources in this space.
4. Dynamic Byte Latent Transformer: A New Approach to Language Models
Most language models rely on tokenization, breaking text into predefined chunks. Meta's Dynamic Byte Latent Transformer takes a different route—processing raw bytes instead.
Why Bytes Matter
Token-based models can stumble on misspellings, rare words, or adversarial inputs. Byte-level models, by contrast, handle raw text more robustly. Meta's 8B-parameter version outperforms traditional models in efficiency and resilience, showing a +7-point average advantage in robustness tests.
By releasing the model weights, Meta invites researchers to explore this alternative, which could lead to more adaptable and resilient AI language systems.
5. Collaborative Reasoner: AI That Works With Humans
The final release tackles one of AI's biggest challenges: collaboration. Humans excel at teamwork, but getting AI to do the same requires more than just problem-solving—it needs social skills like communication, empathy, and feedback.
Building Social AI
Current LLMs aren't trained for multi-turn, goal-oriented collaboration. Meta's Collaborative Reasoner framework evaluates and improves these skills through synthetic interactions where AI agents work together on tasks like math problems or interview prep.
Using a high-performance serving engine called Matrix, Meta generated synthetic data where an LLM collaborates with itself, improving performance by up to 29.4% compared to solo reasoning.
The Bigger Picture
These five releases aren't just isolated advancements—they're interconnected steps toward AI that perceives, reasons, and interacts like humans. From sharper vision to better teamwork, Meta FAIR is laying the groundwork for machines that don't just compute but truly understand.
As these technologies mature, we can expect AI to become more intuitive, adaptable, and capable of seamless human collaboration. The future of AI isn't just about intelligence—it's about creating machines that think, see, and work the way we do.