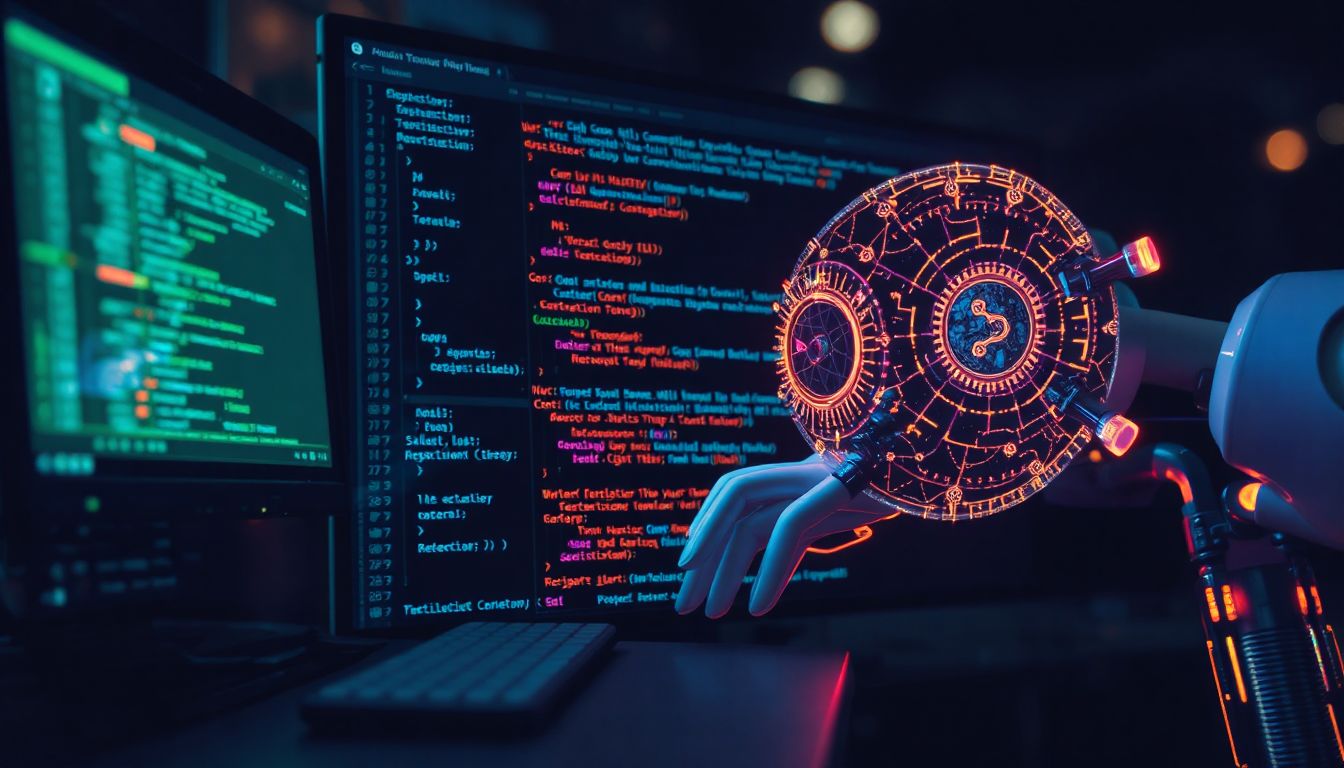
Introduction: The Growing Importance of Securing Gen AI
Generative AI (Gen AI) is transforming industries, from automating customer service to generating creative content. But as businesses rush to adopt these powerful tools, many overlook a critical aspect: security and reliability. Without proper safeguards, your AI investment could become a liability—exposing sensitive data, producing inaccurate results, or even being exploited by malicious actors.
Why Debugging and Data Lineage Matter for Gen AI
Debugging and data lineage aren’t just technical buzzwords—they’re essential practices for protecting your AI systems. Debugging ensures your models perform as intended, while data lineage tracks the origins and transformations of data, helping you verify its integrity. Together, they form a robust defense against risks like hallucinations, data poisoning, and adversarial attacks.
The Risks of Unsecured Gen AI
Imagine deploying a customer service chatbot that suddenly starts sharing incorrect or harmful information. Or worse, a malicious actor tricks your AI into revealing confidential data. These scenarios aren’t hypothetical—they’re real risks businesses face when Gen AI isn’t properly monitored and secured.
Debugging Techniques for Optimal AI Performance
Debugging AI isn’t the same as debugging traditional software. Because AI models learn from data, their behavior can be unpredictable. Here’s how to debug effectively:
1. Clustering for Anomaly Detection
Clustering groups similar inputs and outputs, making it easier to spot anomalies. For example, if a chatbot consistently gives wrong answers to certain questions, clustering helps identify patterns in those failures.
2. Real-Time Monitoring
AI models can drift over time as data changes. Real-time monitoring tools track performance metrics, flagging deviations before they impact users.
3. Root Cause Analysis
When something goes wrong, you need to know why. Root cause analysis digs deep into model behavior, helping teams fix issues rather than just applying temporary patches.
Data Lineage: Tracking the Lifecycle of AI Data
Data lineage is like a genealogy report for your AI’s training data. It answers critical questions: Where did this data come from? How was it processed? Has it been tampered with?
1. Ensuring Data Authenticity
Bad data leads to bad AI. Data lineage verifies the sources and transformations of datasets, ensuring your model isn’t learning from corrupted or biased information.
2. Compliance and Auditing
Regulations like GDPR require businesses to explain AI decisions. Data lineage provides a clear trail, making compliance easier and reducing legal risks.
3. Detecting Adversarial Attacks
Hackers can manipulate AI by feeding it poisoned data. Data lineage helps detect these attacks by identifying suspicious changes in data sources or processing steps.
Combining Debugging and Data Lineage for Maximum Security
Used together, these techniques create a powerful shield for your AI investments:
1. Proactive Threat Detection
By monitoring both model behavior and data flows, you can catch issues before they escalate—whether it’s a bug, a drift, or an attack.
2. Faster Incident Response
When problems arise, debugging and data lineage help teams pinpoint the cause quickly, minimizing downtime and damage.
3. Building Trust in AI
Customers and stakeholders need to trust your AI. Demonstrating robust debugging and data lineage practices builds confidence in your technology.
Implementing These Techniques in Your Organization
Getting started doesn’t require a complete overhaul. Here’s a step-by-step approach:
1. Assess Your Current AI Security
Audit existing models to identify vulnerabilities. Are you tracking data sources? Do you have debugging protocols in place?
2. Choose the Right Tools
Invest in observability platforms that support AI debugging and data lineage tracking. Look for features like anomaly detection and real-time alerts.
3. Train Your Team
AI security is a team effort. Ensure your data scientists, engineers, and security teams understand these techniques and how to apply them.
4. Iterate and Improve
AI security isn’t a one-time task. Continuously refine your processes as new threats emerge and your AI systems evolve.
Conclusion: Protecting Your AI Future
Generative AI offers incredible opportunities, but only if it’s secure and reliable. By prioritizing debugging and data lineage, you’re not just fixing problems—you’re preventing them. This proactive approach safeguards your investment, ensures compliance, and builds trust with users. In the fast-moving world of AI, that’s a competitive advantage you can’t afford to ignore.