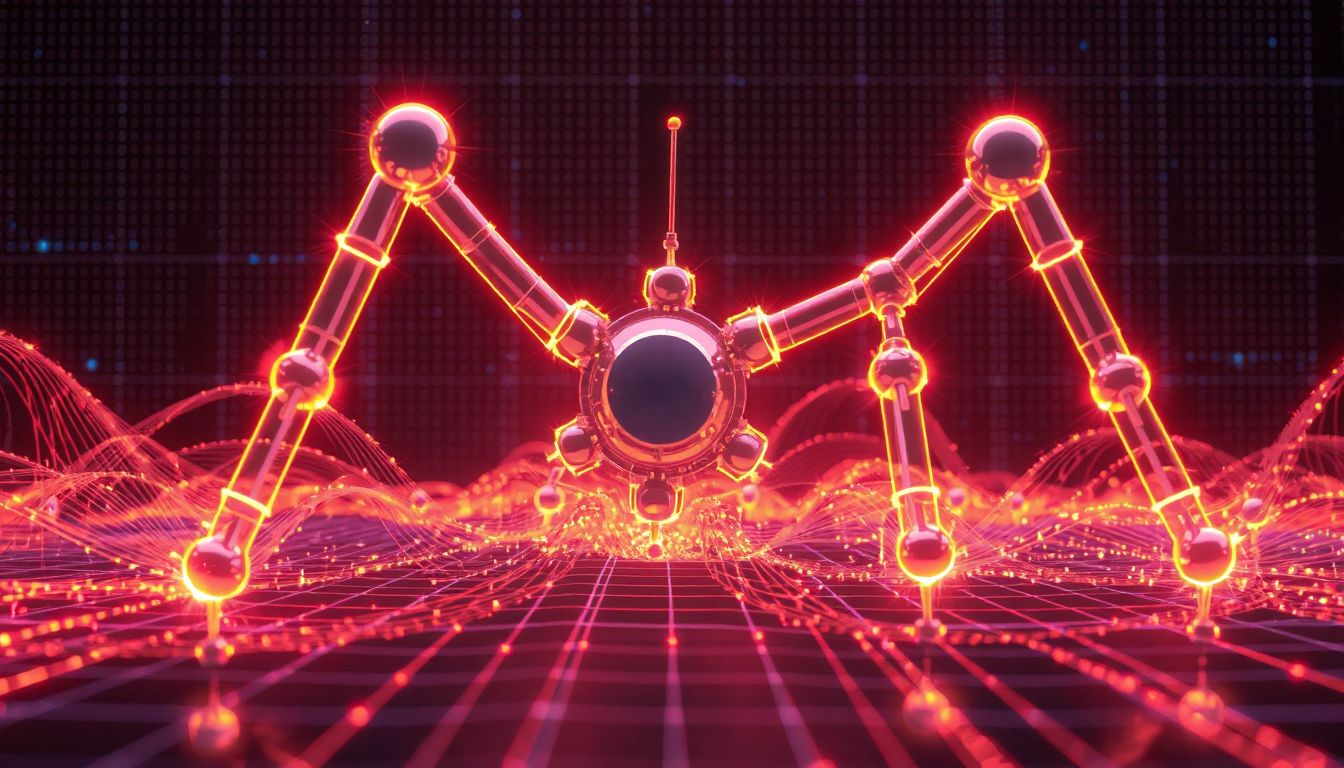
Study Claims OpenAI Trains AI Models on Copyrighted Data: What You Need to Know
Artificial intelligence has been making waves across industries, but a new study is stirring up controversy around how some of these powerful models are trained. Recent research suggests that OpenAI, one of the leading AI companies, may have used copyrighted material to train its models without proper authorization. This revelation raises important questions about ethics, legality, and the future of AI development.
The Shocking Findings About OpenAI's Training Data
According to the study, OpenAI's advanced models, particularly GPT-4, show remarkable familiarity with paywalled and copyrighted content from major publishers. Researchers tested the AI's knowledge against a collection of proprietary books and found that the models could recognize and recall content that shouldn't be publicly available. This suggests the training data may have included material that was never meant for such use.
What makes these findings particularly concerning is that earlier versions of OpenAI's models didn't demonstrate this same level of recognition. The study points to a significant change in how newer models are being trained, potentially crossing ethical boundaries in the process.
Why This Matters for the AI Industry
The implications of these findings extend far beyond just one company. If proven true, this practice could undermine trust in AI systems and create legal headaches for the entire industry. Content creators and publishers have been increasingly vocal about protecting their intellectual property, and this study adds fuel to that fire.
Moreover, the research highlights a growing tension between the need for vast amounts of training data and the rights of content creators. As AI models become more sophisticated, they require more diverse and high-quality data - but where that data comes from matters just as much as what it contains.
The Legal and Ethical Gray Area of AI Training
Current copyright laws weren't written with AI training in mind, creating a legal gray area that companies have been navigating carefully. While some argue that using copyrighted material for training falls under fair use, others contend that reproducing or building upon protected works without permission crosses a line.
How Researchers Uncovered the Potential Copyright Issues
The study employed sophisticated techniques to determine whether AI models had been exposed to specific copyrighted materials. By testing the models' ability to distinguish between original content and paraphrased versions, researchers could gauge how familiar the AI was with the protected works.
One particularly telling finding was that newer models showed much stronger recognition of non-public content compared to material that was freely available online. This discrepancy suggests the training process may have accessed sources beyond what's legally permissible.
The Potential Consequences for Content Creators
If AI companies continue to use copyrighted material without permission or compensation, it could have devastating effects on creative industries. Authors, journalists, and other content producers might see their work being used to create competing AI systems without seeing any financial return.
This could create a dangerous cycle where high-quality content becomes harder to produce commercially, ultimately reducing the pool of quality training data available for future AI development.
The Broader Impact on AI Development
This controversy comes at a critical time for AI development. As governments around the world begin to draft regulations for artificial intelligence, issues like training data transparency are moving to the forefront of policy discussions.
Emerging Solutions and Industry Responses
Some companies are already exploring alternative approaches to training data acquisition. Licensing agreements with content providers and the development of synthetic data generation methods are gaining traction as potential solutions to these ethical dilemmas.
OpenAI and other major players in the AI space will need to address these concerns head-on if they want to maintain public trust and avoid legal challenges. Transparency about training data sources and compensation models for content creators may become necessary steps in the evolution of responsible AI development.
What This Means for the Future of AI
The outcome of this debate could shape the trajectory of AI development for years to come. If stricter regulations are implemented regarding training data, it might slow progress in some areas but could also lead to more sustainable and ethical AI systems.
For now, the study serves as an important reminder that technological advancement shouldn't come at the expense of creators' rights. As AI continues to transform our world, finding the right balance between innovation and ethics will be crucial for building systems that benefit everyone.
Key Takeaways from the OpenAI Copyright Study
1. New evidence suggests OpenAI's models may have been trained on copyrighted material without permission
2. Advanced models show surprising familiarity with paywalled and protected content
3. The findings raise serious ethical and legal questions about AI training practices
4. Content creators could face significant challenges if their work is used uncompensated
5. The industry may need to develop new standards for training data transparency
Looking Ahead: The Path Forward for Ethical AI
As we grapple with these complex issues, one thing is clear: the AI industry needs to have an open and honest conversation about how its models are trained. Whether through voluntary standards, government regulation, or market forces, solutions must be found that respect intellectual property rights while still allowing for technological progress.
The coming months will likely see increased scrutiny of AI training practices, and companies that proactively address these concerns may gain a competitive advantage. For now, this study serves as an important wake-up call about the hidden costs of some AI development methods.